The enterprise world is experiencing a seismic shift as organizations seek to enhance efficiency, innovation, and decision-making through automation and AI technologies. Yet, the future of enterprise AI transcends simple task automation; it demands systems that autonomously evolve and optimize themselves over time. Such systems must not only adapt to changing business needs but also continuously learn, improve, and discover novel solutions without human intervention.
Odin AI stands as a leader in this transformation, advancing the vision of creating AI agents capable of lifelong learning. By leveraging cutting-edge large language models (LLMs), Odin AI’s agents are able to autonomously interact with, learn from, and optimize enterprise operations in real-time. These agents go beyond static AI tools—they act as continuously evolving, embodied systems that can develop new skills, refine existing processes, and make autonomous decisions, all while seamlessly integrating into an enterprise’s ecosystem of tools and technologies.
This capability is inspired by pioneering work seen in projects like Voyager, which demonstrates open-ended learning, and Anthropic’s advancements in contextual retrieval, where AI systems learn by leveraging vast amounts of contextual data. Odin AI embodies these principles within the enterprise context, ensuring that its agents are not merely reactive but proactive, evolving continuously to stay ahead of the curve in increasingly complex business environments.
In this blog, we explore how Odin AI is leading the charge in creating a new era of business operations powered by autonomous, learning-driven agents that continually enhance business efficiency and growth.
Recommended Reading
“Odin AI Task Automator Guide: Simplifying Multi AI Agent Workflows”
What it Means to “Embody” an Agent Using LLMs
To “embody” an AI agent using large language models (LLMs) like Odin AI does, means to combine the language-based reasoning capabilities of LLMs with real-world or simulated physical interactions. Odin AI’s agents are capable of understanding the context of an enterprise environment, reasoning about tasks, and taking actions within that environment. The LLMs serve as the brain, generating sophisticated strategies based on the current state of the system, while the agent’s “body” interacts with enterprise tools and systems to carry out tasks autonomously.
Odin AI agents, powered by LLMs, go beyond performing static tasks—they continuously learn and adapt. For instance, if an Odin AI agent is tasked with optimizing a workflow, it will generate an initial plan, execute actions, monitor the results, and iteratively refine its approach based on feedback from the environment. Over time, it can discover more efficient ways to accomplish its goals, just as a human would.
How Embodied Agents in Odin AI Create a Dynamic, Self-Sustaining Enterprise Environment
Odin AI’s current agents, which automate specific tasks, can evolve into embodied, self-sustaining agents capable of interacting with dynamic enterprise environments. These agents can not only execute tasks but will also learn, adapt, and optimize their actions over time. This transition will enable enterprises to go beyond traditional automation and provide continuous, real-time improvements across various departments.
1. Real-Time Interaction and Adaptation
Odin AI agents can continuously interact with enterprise systems (like ERP and CRM) in real-time, autonomously learning from each interaction. These agents can be configured to adapt dynamically to evolving business environments, whether by analyzing new customer behaviors or reacting to supply chain fluctuations. This means Odin AI agents can be empowered to a level where they can autonomously identify and optimize functions, such as adjusting stock levels or rerouting shipments, making enterprise processes more proactive than reactive.
Scenario
An Odin AI agent monitoring an enterprise’s global supply chain will continuously analyze real-time data, including shipping rates, port delays, and supplier performance. When the agent detects sudden changes—such as rising shipping costs due to geopolitical events or delays at a key port—the system will autonomously take corrective action. Without human intervention, the agent will initiate a series of optimizations, including adjusting procurement timelines, rerouting shipments, and renegotiating supplier contracts.
Example
If a supplier delays the shipping of critical components, Odin AI will not only detect the delay but will also immediately source alternative suppliers. The agent will autonomously initiate a request for a quotation (RFP) from multiple suppliers, assess lead times and costs, and reroute orders to the most reliable vendor. Simultaneously, it will optimize shipping routes, factoring in real-time logistics data like port congestion and customs clearance times. By automatically reconfiguring the procurement process, Odin AI ensures that production timelines are not impacted, maintaining operational continuity. This level of real-time adaptation and proactive decision-making ensures that the supply chain remains resilient and optimized, even in unpredictable global environments.
This enhanced response reduces downtime, cuts costs, and ensures consistent delivery performance, providing a significant advantage over traditional rule-based systems that rely on human input for adjustments.
2. Self-Sustaining Workflow Optimization
As Odin AI’s agents evolve, they will no longer be confined to predefined tasks. The agents will be capable of using self-guided discovery and real-time feedback loops to continuously refine processes. For instance, an agent managing financial reporting will autonomously identify inefficiencies, suggest optimizations, and implement improvements, driving greater efficiency without requiring manual input. These agents will maintain a self-sustaining workflow where each interaction with the environment helps them improve.
Scenario
Odin AI agents will autonomously analyze millions of transactions across multiple departments, leveraging real-time data to flag unusual variances with precision. These agents will detect patterns of discrepancies across financial data—such as recurring anomalies, policy violations, or unusual spending patterns—and proactively suggest adjustments to reporting workflows. By continuously learning from the data, Odin AI will fine-tune its algorithms, ensuring that future financial reports are not only more accurate but also produced more efficiently, minimizing human intervention and manual errors.
Example
During the preparation of a quarterly financial report, Odin AI will autonomously detect recurring anomalies in operating expenses across several business units. For instance, if one unit consistently records outlier expenses in travel and accommodation, Odin AI will identify these patterns, cross-check them against historical data, and flag them for further investigation. Simultaneously, the agent will refine its variance analysis algorithms, taking into account these recurrent anomalies. It will then automate and optimize the entire variance analysis process, adjusting thresholds and parameters to improve accuracy in subsequent reports. This adaptive optimization will reduce manual review time by up to 70%, ensure higher data integrity, and deliver more insightful reports with predictive alerts for potential financial discrepancies in future quarters.
By eliminating the need for manual analysis, Odin AI will free up financial teams to focus on strategic decision-making, ensuring continuous accuracy and efficiency in financial reporting cycles. This proactive approach will not only enhance reporting accuracy but also help detect potential financial risks early, making enterprises more efficient than resilient in their financial operations.
3. Dynamic Learning and Knowledge Expansion
Odin AI’s future agents will build and expand dynamic knowledge graphs by interacting with various data sources. As they gather more data, they will broaden their understanding, applying this knowledge to new and more complex tasks. This continuous learning process will enable Odin AI agents to handle evolving business requirements across different departments, from automating compliance checks to optimizing real-time operations.
Scenario
Odin AI agents managing customer service will continuously analyze historical and real-time customer interactions to learn from past feedback and adjust responses accordingly. These agents will leverage their ability to expand their knowledge graph with every interaction, enabling them to provide more accurate and personalized responses. Over time, Odin AI agents will autonomously enhance their customer service capabilities, improving their understanding of diverse customer queries and offering more tailored solutions.
Example
When a customer submits a query about a product issue, Odin AI will immediately search its knowledge graph for similar queries and solutions, factoring in the customer’s purchase history and past interactions. Over time, the agent will refine its understanding of common issues, autonomously expanding its ability to handle more complex queries. For example, if the agent detects recurring issues related to software bugs, it will proactively flag these patterns, offer tailored solutions, and update its knowledge base to improve future responses.
This continuous learning ensures that Odin AI agents deliver more efficient and personalized customer support, reducing the need for human intervention and improving overall service quality.
4. Self-Optimization Across Multiple Domains
Unlike current AI systems that require retraining, Odin AI agents will self-optimize in the future. They will autonomously refine their performance by learning from the data they process and the outcomes they observe. For example, agents responsible for financial analysis will automatically adjust to changing market conditions or regulations, improving their accuracy and efficiency without needing human intervention or system downtime.
Scenario
Odin AI agents will autonomously manage everyday tasks such as email communication, understanding the context of your role within the company. These agents will analyze incoming emails, learning from your past responses and priorities, enabling them to categorize, prioritize, and draft replies. By continuously adapting to your communication style and workflows, the agents will become more efficient in handling your daily workload.
Example
If you are a department head receiving emails from internal teams, external clients, and vendors, Odin AI will analyze the content and urgency of each email. For example, when an important email from a client regarding contract negotiations arrives, the agent will prioritize it, suggest an appropriate response based on previous communications, and draft a follow-up. It will also handle lower-priority emails, such as internal updates, by providing templated responses or summarizing information for quick review.
Over time, Odin AI will self-optimize across multiple communication domains, such as project updates, client relations, and team management, learning from your habits and preferences. This will allow your enterprise workforce to handle ever-increasing volumes of emails without getting overwhelmed while ensuring critical communication is addressed promptly and efficiently.
5. Continuous Improvement Without Downtime
In the future, Odin AI’s agents will operate in a self-sustaining cycle of continuous improvement. As they evolve, they will be able to adapt to new data and requirements on the fly, ensuring that enterprises benefit from ongoing optimizations without the need for retraining or downtime. This continuous improvement cycle will allow enterprises to become more efficient and resilient, as Odin AI agents will dynamically evolve in response to changing business needs.
The Role of LLMs in Lifelong Learning
LLMs are pivotal in enabling autonomous agents to continuously learn and adapt in dynamic environments. By generating task-specific strategies, analyzing feedback, and iterating on actions, LLMs allow these agents to refine their performance over time. This iterative process mimics human learning, where experience leads to improved skill and adaptability.
The combination of LLMs and embodiment creates agents that can act autonomously, self-correct, and acquire new skills indefinitely, making them highly useful in real-world enterprise settings where conditions are ever-changing.
Recommended Reading
How To Build An AI Agent: A Tech Enthusiast’s Guide
Anthropic’s Contextual Retrieval—Augmenting Lifelong Learning
The Importance of Contextual Retrieval
Contextual retrieval is a technique that enhances an AI’s ability to fetch relevant information by embedding additional context into each data chunk before retrieval. This significantly reduces errors that arise from isolated data retrieval, ensuring that the AI can make sense of a query in its full context. Contextual retrieval also integrates with prompt caching, which lowers processing costs and makes the system more efficient, especially for large-scale applications.
For example, if an AI retrieves a piece of financial data, it might initially fetch a statement such as “The revenue grew by 3%.” However, without understanding the broader context—such as which company or time period is referenced—the response could be ambiguous. Contextual retrieval solves this by embedding additional metadata, ensuring that the AI fetches a fully contextualized response (e.g., “ACME Corp’s revenue grew by 3% in Q2 2023”)
How Odin AI Continuously Learn Through Contextual Retrieval Mechanism?
Drawing from Anthropic’s contextual retrieval techniques, Odin AI uses a similar method to enhance information retrieval from large enterprise knowledge bases. This mechanism allows Odin AI to retrieve relevant data while preserving the context in which the information was originally embedded. By appending chunk-specific context before indexing and embedding, Odin AI can ensure that the data fetched is highly relevant and specific to the query, making its decision-making process more precise and informed.
Contextual Embeddings
Odin AI preprocesses each chunk of information by adding concise, chunk-specific context that helps the AI interpret the data more accurately. This preprocessing significantly reduces the likelihood of retrieval errors, ensuring that each chunk retrieved includes the necessary surrounding context (e.g., time period, location, or entity). This feature enables Odin AI to reduce failure rates in complex information retrieval tasks by up to 35%, leading to more reliable and accurate enterprise data analysis.
This improvement is critical in enterprise environments where Odin AI must handle vast amounts of specialized data like legal documents, financial reports, or operational logs.
BM25 and Embeddings Fusion
To further enhance retrieval efficiency, Odin AI combines semantic embeddings (which capture the meaning of data) with BM25 (which focuses on exact lexical matches). This hybrid approach ensures that Odin AI can retrieve the most relevant and context-specific knowledge with high precision. For example, in enterprise use cases involving legal or financial documents, this combined method reduces retrieval failures by up to 49%, improving the system’s ability to quickly access critical information.
Recommended Reading
AI Agents in 2024: Trends Tech Enthusiasts Should Watch
Scenario-based Examples
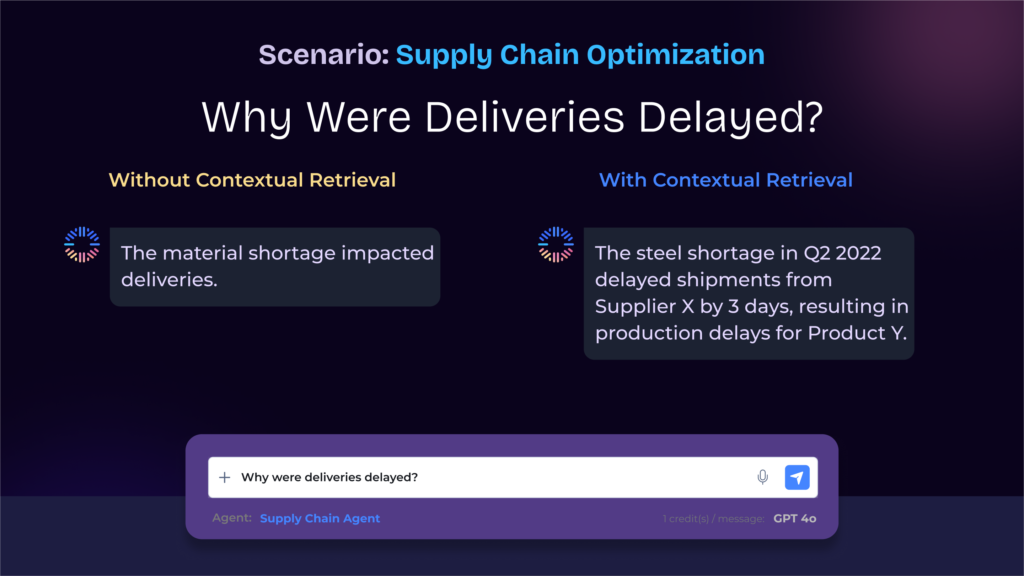
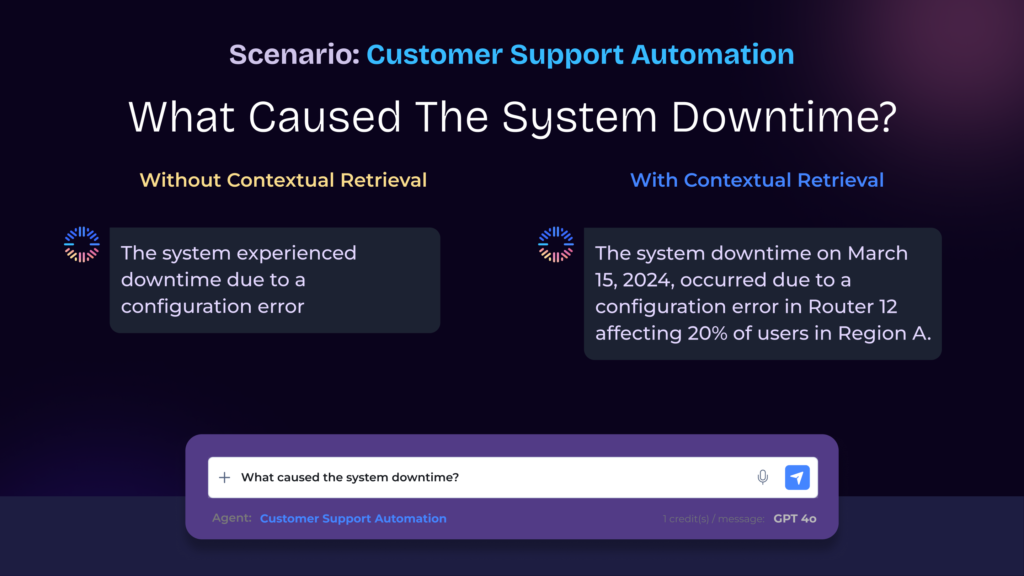
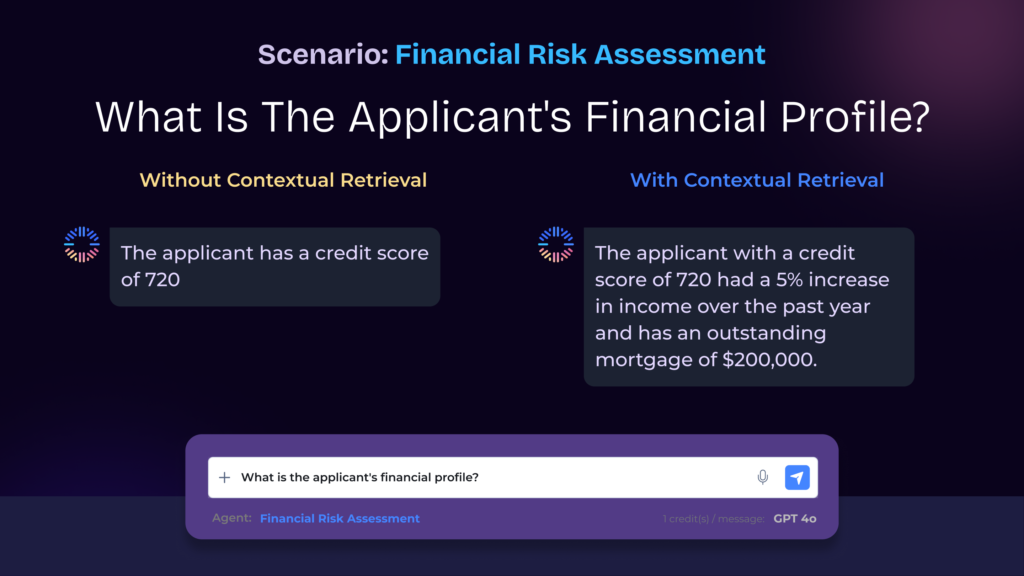
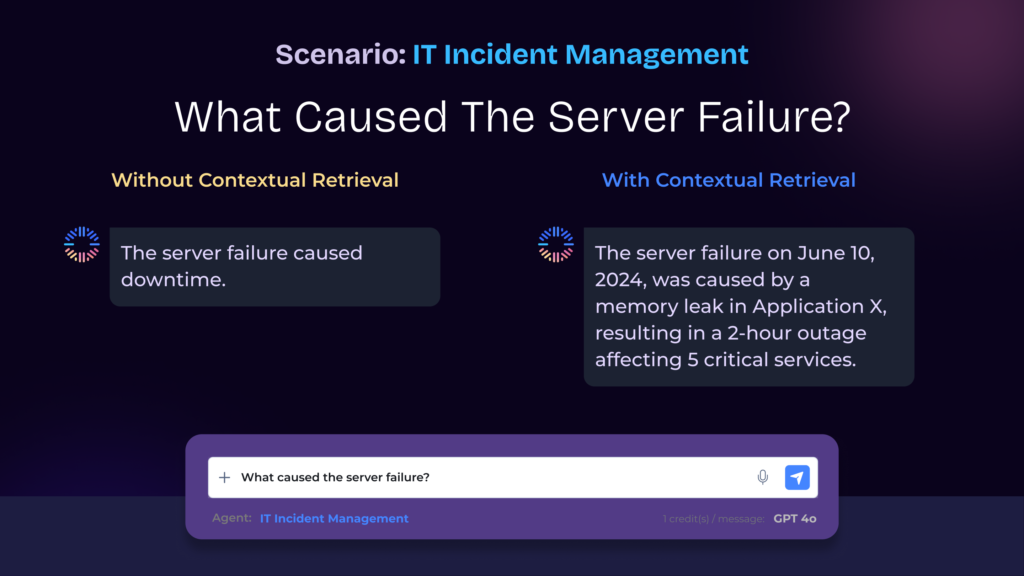
API Integrations for Enterprise Automation with Odin AI
Odin AI agents utilize APIs to interface with numerous enterprise applications, such as financial systems, CRM platforms, and databases. These connections enable the agents to pull relevant data, process it, and execute tasks autonomously. For example, Odin can integrate with MySQL databases to handle real-time data processing, financial analysis, and reporting through SQL agents. This allows the system to continuously gather and analyze vast amounts of data, leading to more efficient workflows and decision-making capabilities.
Example: Integration with Financial Reporting Tools
One powerful use case of Odin AI’s API integrations is in financial reporting. By integrating with financial reporting tools via API, Odin AI automates critical accounting processes such as variance analysis, report generation, and compliance monitoring. For instance, Odin’s Data agents (SQL Agent) can pull financial data from various accounting platforms and ERP systems, automatically analyze it, and generate detailed reports. This automation reduces manual data handling and reporting times from weeks to seconds, allowing finance teams to focus on strategic tasks rather than repetitive work.
Furthermore, real-time data access through APIs ensures that reports are always based on the latest financial data, which is crucial for timely decision-making in fast-paced business environments. These integrations can scale to accommodate growing data volumes, making them suitable for businesses of all sizes.
Recommended Reading
Using AI Agents For Technical Document Search: A Detailed Case Study
The Enterprise OS of the Future
Vision of Odin’s Business OS
Odin AI is laying the groundwork for a future where enterprises will operate on a dynamic, self-sustaining business operating system. This Enterprise OS will consist of multiple autonomous agents working in tandem across various business functions. The agents will continuously learn, evolve, and optimize business processes. This system goes beyond isolated automation by creating a network of agents that can share insights, learn from each other, and collaboratively drive the business toward higher efficiency and innovation.
In essence, Odin AI’s Enterprise OS of the future will be a powerful, adaptable platform for companies that want to embrace automation, scalability, and innovation. With agents collaborating and learning autonomously, businesses will have the agility to grow, adapt, and thrive in increasingly complex environments.
Have more questions?
Contact our sales team to learn more about how Odin AI can benefit your business.
FAQs
Odin AI's Autonomous Lifelong Learning Agents are AI systems capable of continuously learning and optimizing their actions in real-time. They interact with enterprise environments, adapt to changes, and autonomously improve processes across various business domains without needing human intervention.
LLMs power Odin AI’s agents by providing language-based reasoning and decision-making capabilities. The agents use LLMs to understand the context, generate strategies, and execute actions within an enterprise, continuously refining their performance based on feedback from the environment.
Odin AI’s agents autonomously refine their actions by learning from the data they process and the outcomes they observe. This allows them to self-optimize without needing retraining, improving their accuracy and efficiency across domains like finance, supply chain, and customer service.
Odin AI’s agents continuously monitor and optimize business processes in real-time. They implement improvements without requiring system downtime or manual intervention, ensuring that enterprises benefit from ongoing enhancements and operational efficiency.
Yes, Odin AI agents are designed to handle large-scale enterprise functions. In supply chain management, for example, they can autonomously detect disruptions, reroute shipments, and optimize procurement processes to ensure smooth and cost-efficient operations.
Odin AI uses contextual retrieval to ensure that all relevant information is retrieved with the necessary context, reducing errors and improving decision-making. This allows the agents to make more informed, context-aware decisions that enhance their ability to solve complex enterprise problems.